Conducting assessments and appraisals
Before staring an assessment, it is useful to conduct a literature review to draw together information about the country and local areas for planning climate-smart agriculture actions. A literature review can avoid wasting efforts in replicating work that has already been completed and can identify information gaps. Customized assessment complements the literature review by collecting and analysing additional data.
Abundant information on climate change and its impact on agriculture are available at global and regional scales. Information at national and subnational scales is scarcer, but can be found from a range of sources, including:
- the Intergovernmental Panel on Climate Change (IPCC) Fifth Assessment Report;
- the IPCC Special Report on Managing the Risks of Extreme Events and Disasters to Advance Climate Change Adaptation (IPCC, 2012);
- National Communications to the United Nations Framework Convention on Climate Change (UNFCCC);
- National Adaptation Programmes of Action (NAPAs);
- national climate change strategy and action plans;
- academic papers;
- non peer-reviewed reports from national ministries, climate change bodies, and research institutions and universities.
The major steps for designing assessments and appraisals can be summarized as:
- conduct literature review;
- identify stakeholders;
- assess information needs of stakeholders;
- evaluate of the roles and capacities of stakeholders; and
- design assessments and appraisals, including agreements on climate-smart agriculture objectives by stakeholders.
Once the study design is drawn up in consultation with stakeholders, choices can be made about the data, methods and tools that best meet the specified spatial and temporal scales and the other requirements of the assessments and appraisals. There is a variety of methods, tools and databases for climate data, climate downscaling tools and vulnerability assessment tools (e.g. GTZ, 2009; World Bank, 2009a; UNDP, 2009, 2010; FAO, 2012a). Climate-smart agriculture planners should choose a method that suits the needs of the project and programme. There is no single method that is applicable to all climate-smart agriculture projects.
C8 - 2.1 Conducting climate impact assessments
Climatic context
Climate-smart agriculture is a response to historical and on-going changes in climate and projected climate change. Weather and climate data are key components of all climate-smart agriculture interventions. A correct understanding of local climatic conditions, trends and projections forms the foundation for assessing climate impacts and monitoring and evaluating climate-smart agriculture practices. In a top-down approach, focus should be on climatic variables (Table C8.2) that are related to agriculture, such as rainfall and the intensity, extent, and duration of droughts.
Weather observations are collected at tens of thousands of land-based weather stations around the world. They are complemented by observation by ships, radiosondes, aircrafts and satellites. Some of the data are shared with the international community; other data are owned by the country. At the outset, it is advisable to inquire with the national weather service about data availability. Data availability and quality varies significantly by location, country, climatic variables and temporal frequencies. Observed climate data may be verified and complemented by local knowledge of climate trends.
Table C8.2. Examples of changes in climate with direct or indirect consequences on agriculture and vulnerability
|
Future projections of climate are outputs from global climate models, which typically operate at a coarse resolution of about 100 kilometres x 100 kilometres, or larger. Global data are often translated into finer spatial scales through a process termed downscaling. For more discussions of climate data collection and analysis, consult FAO (2012a), UNDP (2009) and UNFCCC (2010a).
Availability of data and information
All assessment activities require data on a range of subjects, including climate, agriculture, socio-economic conditions, capacities and livelihoods. Box C8.1 illustrates agronomic weather indices developed to help orient adaptation efforts. The quality of data directly affects the quality of assessment. Analyses based on poor quality data are of very limited value.
Natural climate variability can best be described with long-term climate data that covers many decades. Anthropogenic climate change alters climate variability and mean climate. To characterize current and historical impacts of climate change on agriculture for policy and project design, good quality, continuous and long-term climate data (e.g. temperature, rainfall, wind, solar radiation, humidity, evaporation and runoff) from ground observations and satellites are necessary. Along with climate data, agricultural statistics describing the state of agriculture are necessary for the same time span. Such data includes crop yields and areas under cultivation, as well as fish and wood production. However, data availability and quality are often an issue in many developing countries (OECD, 2009; UNFCCC, 2010b; Lamhauge et al., 2011).
Box C8.1 Agronomic Weather Indices
Weather events that occur during the course of a cropping season can have a tremendous impact on crop survival, growth and productivity. At various points in their development (e.g. post-germination, reproduction, yield formation), all crops have varying sensitivities to specific types of weather stresses. Viewed over time, observable trends in the frequency and/or changes in the severity of these intra-seasonal weather events define the principal attributes of climate change to which crop agriculture must adapt (e.g. rising maximum temperatures, shortening of the rainy season, or increased frequency of droughts during the reproductive phase).
To detect these changes, and help orientate adaptation efforts in answering the question “what do we adapt to?” a set of agronomic weather indices have been developed. The indices pair key stages of crop (and crop variety) development with the weather events occurring within each season. Analysis has been carried out in several countries using the national archive of daily weather station temperature and precipitation records. Identifying past trends and projecting these into the immediate future (5 to 15 years), provides a bridge, filling the gap between the empirical past and modelled future. Individually, and in various combinations, results from the analysis have enabled the identification of specific climate trends that require attention, guiding research programmes, extension field activities and development efforts more broadly. The established indices can be used with any crop for which a basic physiological understanding exists (e.g. Casas, 2016), and cover spatial scales ranging from individual weather stations to global coverage. A participatory, non-computer based version of the assessment process has also been prepared (Simpson, 2016).
Future climate projections are produced by global climate models at relatively coarse spatial resolution of 100 kilometres or larger. Downscaled climate information at finer spatial scales is usually not readily available in a format that can be easily used by researchers for assessing policy and project design, and by practitioners for monitoring and evaluating project baselines (OECD, 2009; UNFCCC, 2010b; Lamhauge et al., 2011).
The key point is to identify the most important set of data for assessments, and to keep collecting and analysing them. National systems of data collection can be complemented by local systems and by the knowledge and observations of local people.
Improving information and data collection and availability is a priority in many developing countries. Targeted climate-smart agriculture strategies and interventions need to be based on reliable user-oriented information that includes good quality data, documented vulnerabilities and accurate evidence.
Climate impacts on agriculture
An analysis of whether climate variability and change have an impact (historical, current, and future) on the agriculture sectors should take into account agricultural input markets, food demands, transportation, distribution channels, as well as agricultural production. Impact assessment models are typically physical models (e.g. crops, hydrology, fisheries and forestry) or economic models that are highly specialized and require consultation from experts in the field. The Modelling System for Agricultural Impacts of Climate Change (MOSAICC), for example, is an integrated package of models and tools for facilitating an interdisciplinary assessment of the impacts of climate change on agriculture (see Box C8.2).
A common climate impact assessment of agricultural productivity (e.g. crop yield) follows a top-down approach (see chapter C8-1.1). It requires a sound understanding of current and past impacts of climate change on the agriculture sectors and local perceptions of climate change, as well as the collection of long-term historical data of weather and agriculture. Past climate conditions can be associated with past agricultural productivity to establish causal links and calibrate models. Global climate models can provide future climate projections and can be downscaled to finer spatial scales for application. Once calibrated, models can be used to simulate future impacts of climate change on agriculture with projected climate as an input (see also FAO, 2012a for general methodology). UNFCCC (2010a) provides a review of available agricultural models, including: agroclimatic indices with geographic information systems (GIS); statistical models and yield functions and process-based crop models; and economic models, such as economic cross-sectional models, farm-level microeconomic models, household and village models, and macroeconomic models. These different models may be useful for assessing climate impacts, and the assessment of climate-smart agriculture options, in different contexts.
It should be noted that climate is variable by nature. The weather experienced daily is a combined result of natural climate variability and anthropogenic climate change. Climate change manifests itself in various ways. Temperature will increase everywhere, but the size of increase varies spatially and temporally. Precipitation may change both in intensity and frequency. For example, a decrease in annual rainfall may possibly be accompanied by increased flooding. Anthropogenic climate change adds complexity to natural climate variability. It may be difficult to completely decouple the two for the purposes of assessing impacts of climate change on agriculture (Lamhauge et al., 2011; Hedger et al., 2008). For assessing adaptive options, it is not necessary to do so.
Box C8.2 The Modelling System for Agricultural Impacts of Climate Change (MOSAICC) - an integrated modelling system for assessing the impact of climate change on agriculture
The impacts of climate change on crop production are the result of a combination of factors, including changes in temperature and rainfall regimes; variations in the start and length of growing seasons; carbon dioxide fertilization; pest and disease outbreaks; and water availability for irrigation. Changes in agricultural yields will affect food production and have repercussions throughout the national economy. A multidisciplinary approach is necessary for dealing with these different aspects.
MOSAICC is an initiative by FAO that integrates multidisciplinary models to capture different aspects of the impacts of climate change on agriculture, forestry and hydrology. MOSAICC includes a tool for statistical downscaling of climate projections, two crop models (Aquacrop and WABAL), one precipitation-runoff model (STREAM), one forest landscape model, and one economic model (Computable General Equilibrium). All of these tools and models are incorporated into a unique software architecture. A spatial database is defined to link data and models. The architecture also has utilities to process the data from model to model, and web interfaces that can be used to manage data and simulations.
This integrated system offers a number of advantages. Remote access and the system’s user-friendliness (users do not need to install any software on their own computer) facilitate collaborative work with experts around the world. Computing time is reduced and data conversion and reformatting are taken care of within the system. The system allows data tracking down the succession of experiments and can carry out replicable studies. In addition, the modularity makes it possible to constantly update the system by replacing a model or adding a new module. Finally, the system runs at low cost. It does require maintenance, but all software programs are open access.
The whole system is installed on a central server for use by national experts in specialized institutions of developing countries. The different models within MOSAICC should be run by researchers with the relevant expertise. The experts are trained to use the models.
The system was deployed in Morocco in the framework of the European Union/FAO Programme on global governance for hunger reduction, and in Indonesia, Paraguay, Peru and the Philippines in the framework of the Japan-funded Analysis and Mapping of Impacts under Climate Change for Adaptation and Food Security project. Malawi, Uruguay and Zambia are using the system with the support of Climate-Smart Agriculture and National Adaptation Plans Projects.
Vulnerability assessment
The impacts of climate change on agricultural productivity and other aspects of the agricultural sectors can lead to different repercussions in household income and food security. The vulnerability of livelihood depends on the capacity of local communities to substitute a negatively affected production system with an alternative that could prevent losses in agricultural income, provide subsistence production, or supply food to markets. Vulnerability assessments characterize and identify areas, households or sub-populations that have particularly low livelihood resilience. This assessment can help climate-smart agriculture planners prioritize their actions and target vulnerable communities (e.g. youth, elderly, landless people, and women). Vulnerability assessments also provide the basis for the development of strategies to increase the resilience of livelihoods to climate change.
With the potential impacts of climate change identified from previous assessments, vulnerability can be assessed by evaluating the adaptive capacity of the system using a top-down approach. The bottom-up approach, on the other hand, focuses more on collecting different indicators that would characterize the vulnerability of the agriculture sectors and associated sectors to various risks, including climate change. There are a wide variety of possible indicators, including socio-economic resources, technology, infrastructure, information and skills, institutions, biophysical conditions and equity (Dessai and Hulme, 2004). Climate change and variability is considered as a threat to society and the environment, along with socioeconomic, political and institutional structures and changes. Contextual conditions of the society and environment define their adaptive capacity and vulnerability to specific threats. Some of the common methods of vulnerability assessments are categorized in Figure C8.3.
Figure C8.3. Methodologies, methods and characterizing dimensions of outcome and context vulnerability
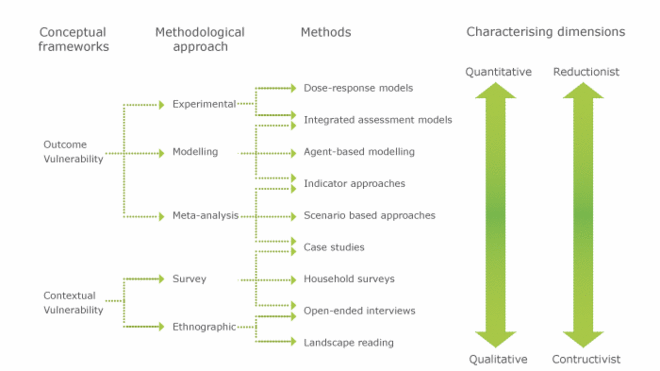
From the perspective of resilience and adaptive capacity, the World Bank (2009b) indicates three broad options for conducting assessments: full scope social assessment and/or extensive consultations (e.g. key informant surveys, focus group discussions, community surveys); rapid social assessments (e.g. checklists of key climate-related vulnerabilities, current coping strategies and limiting factors); and field-testing project management tools (e.g. CRiSTAL). Although not developed specifically for climate-smart agriculture, an empirical resilience model, the Resilience Index Measurement and Analysis (RIMA), developed by FAO (FAO, 2011; Alinovi et al., 2010; Frankenberger et al., 2012), uses structural equation modelling and factor analysis to identify the variables that contribute to household resilience. Common components (and associated indicators) that are considered in the resilience model are: income and food access, access to basic services, social safety nets, assets, adaptive capacity and stability.
Box C8.3 presents, the sustainable livelihood framework for assessing community resilience to climate change.
Box C8.3 Sustainable livelihood framework for assessing community resilience to climate change
A community’s ‘resilience’ to climate change can be assessed using the sustainable livelihood framework. The sustainable livelihood framework shows the relationships between household assets, their vulnerability and the institutional context, which determine household livelihood strategies and their outcomes. The asset pentagon lies at the core of the sustainable livelihood framework. It visually presents information about the type and level of assets the community possesses. The pentagon consists of five different types of assets or capitals: human, social, natural, physical and financial (DFID, 1999).
In general, it can be said that households with fewer assets are more vulnerable to external shocks. However, it is not only the limited amount of assets that matter in measuring people’s coping capacity. Other factors are also important, including: the quality of the assets; whether and how people have access and rights to the resources; whether and how they can use them; and whether and how the resources are or can be shared. Moreover, the institutional context, which consists of policies, institutions and processes, can also influence people’s access to assets and the range of livelihood strategies available to them. Assessing changes in these assets can help to determine a household’s resilience to external shocks. An Assessments of Impacts and Adaptations of Climate Change project in Sudan, for example, used the sustainable livelihood framework to measure the impact of project interventions on a community’s resilience. For each type of capital, a set of criteria and indicators were developed (Elasha et al., 2005). Assessing whether CSA activities have strengthened communities’ resilience to climate change can be undertaken using the sustainable livelihoods framework.
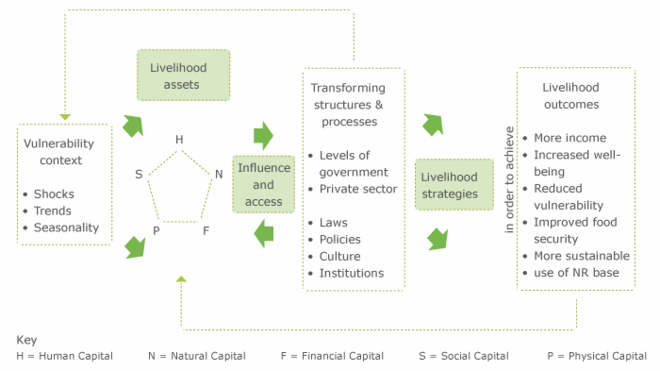
The Self-evaluation and Holistic Assessment of Climate Resilience of farmers and Pastoralists (SHARP) tool is designed as an instrument to assess the resilience of farmer and pastoralist households to climate change. It has three phases. Phase one involves a survey-based evaluation of households’ climate resilience with 13 indicators adapted from Cabell and Oelofse (2012). In phase two, the gaps and areas for improvement in the response of farmers and institutions to climate variability are analyzed. In phase three, the information gathered through the first two phases is integrated with broader analysis of climatic data, with the aim of assisting farmers in prioritizing actions to build the resilience of their agricultural ecosystems, and orienting institutions towards the best possible policy approaches to strengthen climate resilience.
Understanding risk
Risk related to climate impacts is the interaction between climate-related hazards (both extreme events and long term trends), vulnerability (as described above) and exposure of human and natural systems. An illustration of this concept can be found in the Contribution of Working Group II to the IPCC Fifth Assessment Report (IPCC, 2014, Chapter, 19, Figure 19-1). Drivers of hazards, exposure and vulnerability include changes in the climate system, as well as changes in socio-economic processes, including climate change mitigation and adaptation actions. Understanding risk is important because it incorporates the probability of the occurrence of hazardous events or trends with the impacts if they occur. Risk increases as a results of both the high hazards or high vulnerabilities of the societies and systems exposed.
Box C8.4 Climate-risk assessment to reduce the vulnerability of livelihoods
Central Guinea is dominated by the Fouta Djallon Highlands, a large plateau with altitudes ranging between 600 to 1 500 metres and an annual rainfall of 1 800 millimetres. It is the source of a significant number of important rivers on which many West African countries depend. The plateau is threatened by a range of impacts associated with climate change. The Guinea NAPA has reported a decline in annual rainfall, the disruption in normal precipitation patterns and a downward trend in water available from rivers. These meteorological trends have had visible impacts during the last decade: rivers and land are drying out; forests are having difficulty recovering; and agricultural production is being affected.
The Global Environmental Facility Fouta Djallon Highlands Integrated Natural Resources Management Project implemented in Guinea and its neighbouring countries, was designed to ensure the conservation and sustainable management of the natural resources to improve the livelihoods of rural populations directly or indirectly connected to the highlands. The project highlighted the importance of reducing rural communities’ vulnerability to climatic disturbances. However, a clear understanding of the current impact of climate variability was necessary for the project to ensure that the activities would have a positive impact on local resilience to climate change.
Accordingly, a climate-risk assessment of the target communities was undertaken to evaluate the impact of current climate-related hazards on local livelihoods in different agro-ecological zones. During community consultations, farmers reported observing changes in weather patterns over the last decade. These changes included increased frequency of droughts, extreme heat, delays in the arrival of the rainy season, and scarcity and unpredictability of rains. These perceptions about the changes in the timing, intensity and frequency of climatic hazards are consistent with scientific data. Local perceptions on the connections between climate and livelihoods, as well as current and potential coping strategies were discussed and analysed. The appreciation of how the farmers respond to the climatic risks and the identification of resources that are both sensitive to climatic risks and crucial for implementing the coping strategies helped to incorporate climate-smart practices into the project activities. Originally planned activities were adjusted according to the way project activities could affect the availability of critical livelihood resources and the access of local communities to these resources. The purpose of the revisions was to make the project’s activities more resilient to current climate variability and deal with emerging climate issues.
Working with uncertainties
The climate system has its own natural variability, which can be observed at different time scales: daily, seasonally, inter-annually, decadal, centurial. For the near future, natural climate variability may well be far larger than long-term changes in climate caused by increased atmospheric concentration of greenhouse gases. Data collected at weather observation stations are the basis of all climate science, but these stations are few in number and the data quality is not always reliable, particularly in sub-Saharan Africa.
Future climate projections carry complex, multilayered uncertainties. All climate projections are based on emission scenarios that represent a range of possible socio-economic development pathways. It remains uncertain, however, how human activities will evolve in the coming decades and which activities will drive greenhouse gas emissions and influence climate change. Humanity’s ability to adapt to future climatic conditions, the costs of adaptation and the speed at which new technologies will be adopted are not known and can only be considered in scenarios.
Scientific understanding of the climate system also has uncertainties. Even for the same greenhouse gas concentrations, different climate models project different future climates, even though all of the models are known to reasonably reproduce past climate conditions.
Assessments of impacts and characterizations of vulnerability (climate impact assessments) are conducted with a variety of models using climatic information as inputs. A long chain of scenarios and models will indicate the potential local impacts of climate change on agriculture and the possible adaptation responses. But each component of the chain carries its own uncertainties, which may result in a growing number of permutations and an expanding envelope of uncertainty (OECD, 2009; Hedger et al., 2008, World Bank, 2010; Villanueva, 2010; GIZ, 2011a).
Uncertainties associated with climate predictions are an additional consideration on top of the uncertainties related to non-climatic factors that are common in all agricultural development projects.Uncertainty is inherent in the climate-agriculture system and cannot necessarily be reduced through scientific advances. Uncertainty should be recognized and managed. It is not an excuse for delaying action. Where possible, a wise approach is to keep track of uncertainties and be flexible in planning adaptation strategies that reflect the range of projected scenarios. Rather than proposing an optimal policy, taking such an approach will help minimize the vulnerability of an action to a range of possible risks. As climate conditions evolve over the project and programme cycles, and new information about impacts of and vulnerability to climate change becomes available, adjustments may need to be made to the assessments of baseline projections, climate change impacts, climate-smart agriculture measures and indicators for monitoring and evaluation (e.g. GIZ, 2011a).
Adaptation processes need to be designed in response to evolving climate conditions. The carbon balance of the ecosystem is dynamic and may change over time in the absence of mitigation interventions. Project managers may need to adjust the climate-smart agriculture interventions according to revised baseline projections at the project mid-cycle and evaluate the project’s benefits against the new projections. The World Agroforestry Centre (ICRAF) and CGIAR Research Program on Climate Change, Agriculture and Food Security (CCAFS) baselines data collection activities described in Boxes C8.5 and C8.6 provide robust information for making policy and programme comparisons over various timespans.
Box C8.5 Land degradation surveillance baseline framework
ICRAF’s land degradation surveillance framework (LDSF) establishes a biophysical baseline at the plot and landscape levels. It also provides a monitoring and evaluation framework for assessing the processes of land degradation and the effectiveness of rehabilitation measures over time. The LDSF collects information on land use, vegetative cover, soil properties and topography using hierarchical field survey and sampling protocols. The FAO Mitigation of Climate Change in Agriculture (MICCA) Programme has implemented the LDSF in East Africa as a tool for measuring the baseline for land health in pilot projects. There are three additional primary data collection activities (greenhouse gas emissions and agricultural productivity, carbon balance, and socio-economic indicators) to monitor changes in socio-economic and environmental conditions.
The figure below shows an example of LDSF hierarchical sampling design used to establish baseline soil and vegetation information. Ten subplots (red points) are selected at random from 16 clusters within a 10 x 10 kilometres.
Figure C8.4. LDSF hierarchical sampling design
- Source: Adapted from Vågen et al., 2010
Box C8.6 The CCAFS baseline
CCAFS baseline is implemented on three different levels: household, village and organization. It collects indicators that describe current practices and behaviours in relation to livelihood systems and farming practices in CCAFS sites over time. These indicators also indicate changes made to agriculture and natural resources management strategies in the recent past. Other indicators help CCAFS to understand the enabling environment that allows for these practices and behaviors (e.g. natural resource conditions, policies, institutions) and the agricultural and climatic information that organizations that work at each site receive. The objective is to capture diversity across communities and households with sufficient precision to capture changes that occur over time.
The key aim of the CCAFS baseline is to provide snapshots of current behaviour at the sites using instruments that can be applied in all the CCAFS regions. The plan is to revisit the same households and communities after five years, and again in ten years, to monitor the changes that have occurred since the baseline was carried out. The same survey is being conducted in diverse locations across all CCAFS target regions. To date, close to 4 500 households have been surveyed in over 220 villages. In 16 CCAFS sites, 16 communities participated in qualitative focus group discussions, and over 160 organizations have been interviewed at these sites. This has allowed for valid and robust cross-site and cross-regional comparisons. As a result, the baselines are broad rather than deep. The intention is that complex relationships will be explored through further research in the same locations with secondary data.
The emphasis on carrying out cross-site comparisons has two costs. First, the baselines include information on the site’s characteristics, but they are typically not sufficiently detailed for some activities (e.g. farming systems studies). Second, the baselines do not contain all the information needed to do ex-post impact assessment studies. Such studies are usually designed to evaluate specific technological or policy changes in a location and attribute the changes to particular activities carried out by specific agents. The CCAFS baseline meets the first objective of impact assessment (tracking change over time), but does not allow for the attribution of these changes to specific activities. The goal is not to attribute these changes to the programme, but to assess what kinds of changes have occurred and whether these changes are helping households adapt to, and mitigate, climate change.
All CCAFS baseline guidelines and tools for data collection, processing and analysis, as well as the data itself and the reports are being made publicly available.
For more information, visit the CCAFS web site.
All assumptions that are made in the assessment process and the sensitivities of these assumptions should be explicitly stated and communicated. This will help practitioners interpret the results of assessments for policy formulation and investment design and allow them to better deal with uncertainties as they arise.
When there is no reliable information on which to base climate-smart agriculture decisions (even after carrying out the appropriate assessments for policy and project design, including a cost-benefit analysis), a project may adopt a general ‘no regrets’ approach. Such an approach brings benefits regardless of the size and direction of climate change (or even in the absence of anthropogenic climate change).
C8 - 2.2 Conducting appraisals of climate-smart agriculture options
Evidence regarding the potential benefits of climate-smart agriculture options in terms of enhanced productivity, adaptation, and mitigation can either be built ex ante using simulation models or be produced through a pilot project with a carefully designed biophysical and socio-economic evaluation system to guide subsequent scaling up in a larger climate-smart agriculture programme.
Agricultural productivity and income
Climate-smart agriculture practices should provide an overall strategy on how to achieve sustainable increases in productivity that enhances environmental sustainability and food security. Additional assessment criteria that address a specific sustainability or food security concerns may need to be added to vulnerability and adaptation assessments. Standard literature is available on approaches for food security assessments in agriculture (e.g. FAO, 2009; IFRC, 2006; USDA, 2002).
When evaluating the effectiveness of climate-smart agriculture interventions within an investment framework, and their contribution of these interventions to building clear evidence for delivering benefits, there are several methodologies to determine sustainability contributions, such as household-level socio-economic studies, economy-wide models, scientific trials or biophysical and structural models.
Trials and socio-economic analysis
Carefully designed scientific trials on experimental plots or in laboratories provide valuable information on the potential productivity outcomes of field and farm level practices (e.g. cereal-legume intercropping, improved feed management for livestock). However, to assess the potential outcomes of these practices on sustainability or food security at the national level, they should be ideally combined with larger-scale socio-economic analyses where current and potential management conditions can be incorporated.
Thanks to an improved availability of nationally representative household surveys (e.g. LSMS-ISA, National Crop-Forecast/Post-Harvest Surveys, Agricultural Census) and high-resolution data on climate variables, socio-economic studies are increasingly able to assess a large set of field and farm-level practices controlling for critical weather variables, agro-climatic shocks and other disturbances (Di Falco et al., 2011, Arslan et al., 2015, Asfaw et al., 2016a, 2016b). It is important to ensure that there is enough cross-sectional heterogeneity in the data used for these types of studies to cover areas with different exposure, vulnerability and adaptive capacity profiles.
The costs, both in terms of money and time, of setting up proper quantitative studies to evaluate impacts of climate-smart agriculture interventions may be prohibitive in many cases. A more feasible socio-economic analysis tool may be panel data methodologies (i.e. same households observed over multiple years) to control for unobserved variables that affect sustainability or food security outcomes (Wooldridge 2002). The issue stems from the fact that households choose to adopt certain practices and strategies based on observable characteristics (e.g. age, education, wealth) and unobservable characteristics (e.g. skill, curiosity, risk tolerance and ability). For this reason, the observed outcome cannot be directly linked to the use of a practice, if these other factors are not controlled for. This confounding factor can be removed using panel data methodologies or instrumental variables approaches for clearer attribution (Arslan et al., 2015; Asfaw et al., 2015; FAO 2016a). In the absence of panel data, careful quasi-experimental methodologies in large-scale household data can also be used to assess the impact of one practice on outcomes relevant for sustainability or food security (Kassie et al., 2010), although establishing attribution is harder in these analyses.
Socio-economic studies that are based on econometric analysis of household data are also able to assess how interactions between practices and climate variables affect outcomes; analyse livelihood strategies, as well as field and farm-level practices, that contribute to sustainability; and provide valuable information about how institutions (e.g. social safety nets, input subsidies, weather information services) and many other socio-economic variables, including gender, shape these outcomes (Arslan et al., 2015; FAO, 2015; FAO, 2016b). Once the analyses are conducted, controlling for the effects of relevant shocks, the results can be combined with localized climate change projections to simulate how the productivity contributions of analysed options may be expected to change in the future. This exercise would also contribute to providing information about the sustainability of the documented benefits under various scenarios by combining actual evidence with simulation results.
As part of climate change and food security project, Analysis and Mapping of Impacts under Climate Change for Adaptation and Food Security (AMICAF), an econometric analysis of the changing vulnerabilities of farming households to food insecurity was carried out using existing household survey data in Peru, Indonesia, Paraguay and the Philippines. The approach identified the impact of climate shocks on household-level agricultural productivity and the threats posed by climate risks and other risks to a household’s current and future food insecurity. The analysis resulted in a recommendation of a set of policy instruments that may be able to reduce the impact of climate risks most effectively.
Ex ante simulations
Socio-economic studies based on econometric analysis of household data can only assess practices and strategies that are already implemented by households and can be identified in existing data sources. Assessing the sustainability or food security contributions of new innovative practices and strategies would require simulation models using economy-wide (general or partial equilibrium). Examples of these simulation models include:
- Asia-Pacific Integrated Model (AIM);
- Environmental Impact and Sustainability Applied General Equilibrium model (ENVISAGE);
- Future Agricultural Resources Model (FARM);
- Global Change Assessment Model (GCAM);
- Global Biosphere Optimization Model (GLOBIOM);
- International Model for Policy Analysis of Agricultural Commodities and Trade (IMPACT);
- Modular Applied General Equilibrium Tool (MAGNET); and
- Model of Agricultural Production and its Impact on the Environment (MAgPIE).
Micro-simulations can also be used to analyse the effect of an intervention on production, incomes or other welfare outcomes at more aggregate levels. The global economic models listed above commonly use biophysical cropping system models. Examples of these cropping system models include:
- Agricultural Production Systems sIMulator (APSIM) (Keating et al., 2003)
- Environmental Policy Integrated Climate (EPIC) model (Williams, 1990)
- Decision Support System for Agrotechnology Transfer (DSSAT) (Jones et al. (2003)
- Lund-Potsdam-Jena managed Land (LPJmL) model (Bondeau et al., 2007)
- Nutrient Use in Animal and Cropping systems – Efficiencies and Scales FARM SIMulator (NUANCES–FARMSIM) (van Wijk et al., 2009).
These models, which simulate future crop and livestock productivity under various climate and socio-economic scenarios, can also simulate the impact of new practices on crop growth or livestock productivity.
More recently, structural approaches that bring together economic and biophysical models are being used to provide a more detailed understanding of the combined effects of climate stresses and future socio-economic trends, such as price effects on trade balances, and input re-allocations in production (Nelson et al. 2014; Fernandez and Blanco, 2015). These models can also be used to analyse the food security implications of technologies used in climate-smart agriculture (Islam et al., 2016).
In addition to the micro- and macro-level analyses required to build evidence for the food security and sustainability benefits of climate-smart agriculture options, complementary meso-level analyses are needed to understand the impacts of interventions in the food system. These can include the improvements in value chains, infrastructure and access to credit, insurance and information.
Climate change adaptation
The appraisal of climate-smart agriculture options for adaptation effectiveness are an extension of climate impact assessments. Having gained an understanding of potential impacts of climate change and vulnerability, the best climate-smart agriculture practices for local conditions can be reviewed and identified. Ideally, stakeholders are involved in validating the findings of the assessment and help define and select suitable and workable adaptation options. Process-based crop growth models at the farm level could be used to suggest better management practices to improve yields. Economic models could simulate, for example, the effect of a fertilizer subsidy on productivity, market prices and farm income.
In terms of availability of freshwater resources, water accounting through remote sensing, by confirming estimates of the physical volumes and flows of water in the environment, can assess the extent to which water productivity increases impact water users. The impacts of climate change can be assessed and monitored by analysing possible increased variation or particular trends in these flows. The economic value of water services will be assessed through a cost-benefit analysis.
A screening analysis is a simple method in which the assessor answers yes or no to questions about the desirability or interest in adaptive options. Those options with the most 'yeses' can be given the highest priority or be further assessed using more quantitative analytical methods. Examples of climate-smart agriculture practices and their benefits are laid out in Table C8.3. In multicriteria assessments, stakeholders identify the criteria to be used in assessing adaptations. Common metrics are defined to measure the criteria. Assessors rank each adaptation option against each criterion by giving scores. In cost-effectiveness analysis, the relative costs of different adaptation options that achieve similar outcomes are compared (UNFCCC, 2010b).
There are a number of other prioritization tools for climate-smart agriculture prepared by different organizations, including Climate-smart Agriculture 101 by CCAFS; the Climate-smart Agriculture Profiles by the World Bank and the climate-smart agriculture compendium (see box C10.1 in module C10) on field level practices. These tools emphasize the importance of stakeholder participation and dialogue, and provide quick approaches for ranking different practices. Most of the practices in these toolkits, however, are implemented at the field level and can only be one part of a national level prioritization exercise for a climate-smart agriculture strategic framework, which should include other interventions, such as improvements in national agro-meteorological information systems, disaster risk management, value chains and the food system at large, as well as institutions to support climate-smart agriculture. Given the broader set of options that need to be considered at the national level, the stakeholder consultations need to be designed to ensure the participation of all stakeholder groups.
Table C8.3. Examples of climate-smart agriculture practices and their benefits towards climate change adaptation and mitigation, and food security
- Reduced soil erosion and improved nitrogen efficiency from minimum tillage, cover crops and improved rotations;
- Improved water availability from soil and water conservation activities;
- Improved crop yield with new varieties, changes in farm management (e.g. change in planting techniques, fertilizer, irrigation water use) or short-term weather and climate forecasts;
- Improved livestock productivity through enhanced breeding and feeding practices;
- Improved economic resilience from crop and income diversification; and
- Reduced greenhouse gas emissions or increased soil carbon sequestration through diversified or more efficient management practices.
Economic cost-benefit analyses at the field, farm or household level can be employed to highlight the potential high costs that may prevent adoption (e.g. initial high labour requirements of preparing planting basins, high initial investment costs in presence of credit constraints). It is also important to pay attention to opportunity costs, which are often overlooked in analyses. To ensure the results are representative, household and field-level analyses this may require data collection that specifically covers all the relevant costs of practices and strategies considered under various farming systems and under a range of socio-economic and agro-ecological conditions. Simple cost-benefit analyses provide an overall understanding of how costs and benefits compare on average under different systems,
However, attention needs to be paid to not confuse the results of these analyses, which are average values for the system and socio-economic and agro-ecological conditions, with causal interpretation. Ideally these types of analyses should be combined with econometric analyses to control for a large set of variables that shape decisions and outcomes to validate the results of average benefit-cost analyses (Branca et al., 2016).
In supporting the analytical approaches above, evidence need to be amassed in the field through experiments and trials, as part of climate-smart agriculture projects that compare the effectiveness of different farming practices in a controlled manner.
Climate change mitigation
An assessment of mitigation benefits simulates the dynamics of greenhouse gas emissions and carbon sequestration of climate-smart agriculture options, and quantifies their mitigation potentials. When assessing mitigation potential of climate-smart agriculture practices, the bottom line indicators are the reduction of greenhouse gas emissions and the sequestration of greenhouse gases in the soil and biomass, which is measured using the indicator, tonnes of carbon dioxide equivalent. The greenhouse gases that are of particular relevance for the agriculture sectors are carbon dioxide, nitrogen dioxide and methane.
The effects of climate change mitigation can be quantified following two general approaches: top-down and bottom-up. A top-down approach estimates greenhouse gas emissions in the context of the national economy and uses economic models. By estimating the demand for agriculture-related products and the manufacturing costs using various technologies, these models produce projections of production levels and technologies used for this production, which can be translated to emission levels.
The bottom-up approach uses project-level models to estimate the specific mitigation effect of the activity or policy being implemented. When reporting national greenhouse inventories, the bottom-up approach is used. Monitoring and evaluating emissions as part of project and programmes are discussed in module C9.
Approved methodologies to quantify greenhouse gas emissions and removals
Mitigation in the agriculture sector is measured following the IPCC methodology as detailed in the 2006 IPCC Guidelines for National Greenhouse Gas Inventories. Three IPCC methodologies are relevant when estimating the effect of climate-smart agriculture.
- Generic IPCC methodology: The generic IPCC methodology multiplies activity data with an emission factor, resulting in greenhouse gas emissions or removals.
- Gain and loss methodology: The gain and loss methodology estimates the net carbon stock change (e.g. of biomass) by adding all the gains and losses of carbon stock during the relevant time period. This methodology requires comprehensive inclusion of all carbon gains and losses to be effective. It is used as a default method for forestry activities.
- Stock difference method: The stock difference method estimates carbon stock change by comparing the carbon stock in two points in times (e.g. the status of soil organic carbon). The resulting carbon stock change is then divided by the considered time period, to derive a constant annual carbon stock change value for the period. This methodology is usually used for non-forestry land use, such as cropland, and grassland, as well as for land-use change.
Methodological tiers
The IPCC guidelines have three tiers for methodologies quantifying mitigation. Generally, higher tiers both improve the accuracy of the estimate and increase the effort required for collecting information.
Tier 1 approach uses IPCC default emission factors, which are applicable worldwide, in the IPCC methodology. Tier 1 default values are general conservative, with high levels of uncertainty, and are commonly used only for rough estimation of the effect of a policy or activity. For a realistic estimation, (e.g. a national inventory), a higher tier approach is needed. In combination with data on the type and scale of agricultural practice and technology use, the Tier 1 approach facilitates the estimation of greenhouse gas impacts without the requirement of complex input data. Examples of greenhouse gas calculator tools that provide a guided interface to applying Tier 1 methodologies include:
- The Ex-Ante Carbon-balance Tool (EX-ACT) (see Box C8.7 (Bernoux et al., 2010; Bockel et al., 2013; Grewer, Bockel and Bernoux, 2013)
- The Carbon Benefits Project Tool (Milne et al., 2010; UNEP, 2013)
- The AFOLU Carbon Calculator (Winrock International, 2013);
- The Cool Farm Tool (Hillier et al., 2011);
- Marginal Abatement Cost Curves (FAO, 2012b); and
- Agriculture and Land Use National Greenhouse Gas Inventory Software (Colorado State University, 2013).
These tools are not specific to any region and can be applied in any location.
Box C8.7 EX-ACT: assessing the mitigation potential in agriculture and forestry
EX-ACT provides ex ante estimations of the impact of agriculture and forestry development projects on greenhouse gas emissions and carbon sequestration. EX-ACT is a land-based accounting system, measuring carbon stocks and stock changes per unit of land, expressed in tonnes of carbon dioxide equivalent per hectare and year. The ex ante carbon balance appraisal guides the project design process and decision-making on funding aspects and complements the usual ex ante economic analysis of investment projects. EX-ACT has the potential to support project designers in selecting project activities with higher benefits both in economic and climate change mitigation terms. EX-ACT outputs can also be used in financial and economic analysis.
EX-ACT has been developed based on the IPCC Guidelines for National Greenhouse Gas Inventories and makes use of other existing methodologies. The default values for mitigation options in the agriculture sector are mostly from IPCC (2007). EX-ACT consists of a set of linked Microsoft Excel sheets into which project designers insert basic data on land use and management practices foreseen under the projects’ activities. EX-ACT adopts a modular approach with each module describing a specific land use. It follows a three-step logical framework:
Figure C8.5. Modular structure and outputs of EX-ACT
- A general description of the project (e.g. geographic area, climate and soil characteristics, duration of the project);
- Identification of changes in land use and technologies foreseen by project components using specific modules (e.g. deforestation, forestation, forest degradation, annual and perennial crops, rice cultivation, grasslands, livestock, inputs, energy); and
- Computation of the carbon balance with and without the project using IPCC default values and, when available, ad-hoc coefficients.
The main output of the tool consists of the carbon balance resulting from project activities. EX-ACT is an easy, cost-effective tool that requires a minimum amount of data. It includes resources (e.g. tables and maps) that can help users find the information required to run the model. While, mainly used at the project level, the tool can easily be scaled up and be applied at the programme and sector level. EX-ACT has already been applied to assess agricultural policies and value-chain driven changes in farming systems.
- For more information, visit the EX-ACT website (FAO, 2012c)
Tier 2 approach uses country specific emission factors, or even subregional emission factors, and applies them in the IPCC methodology. It results in lower uncertainty of the calculated greenhouse gas emissions and sequestration. The emission factors are determined through a national/regional measurement effort, which could be a survey, direct measurements or remote sensing analysis. Alternatively, it can be taken from recognized databases that collect verified emission factors. Examples of Tier 2 methodologies are the Global Livestock Environmental Assessment Model (GLEAM) (FAO, 2016c) or the statistical approach to to nitrous oxide and nitric oxide emissions by Stehfest and Bouwman (2006).
Tier 3 approach uses methodologies and emission factors developed specifically for national circumstance. It is the most accurate approach for estimating greenhouse gas emissions and sequestration, but it requires considerable effort to develop and maintain. The methodologies are not automatically transferable to other national circumstance. For this reason, Tier 3 approaches are not generally for climate-smart agriculture initiatives. Examples of Tier 3 methodologies include:
- DayCent (Del Grosso et al., 2002);
- DNDC (Li et al., 1992);
- Roth C (Coleman and Jenkinson, 1996); and
- The Modeling Organic Transformations by Microorganisms of Soils (MOMOS) (Pansu et al., 2009).
While there are efforts to make tier 3 methodologies more user-friendly (e.g. COMET-Farm tool), the majority of greenhouse gas estimations used for policy planning and national reporting rely on Tier 1 methodologies.
Other methodologies
Beyond the UNFCCC mechanisms dealing with mitigation, various other financial organizations and programmes support mitigation activities and use their own methodologies to estimate mitigation potential results. Various international finance institutes are quantifying the mitigation potential of their projects to establish financing criteria. Their methodologies for quantifying mitigation are specific for their needs and their available information, and must be considered specifically for projects seeking finance by these institutes.
There are other climate financing mechanisms, such as climate bonds and voluntary emission reduction certifications, which accredit activities promoting mitigation that comply with the mechanism’s requirements. These mechanisms either apply their own methodologies, or roughly follow the IPCC methodologies in part or whole.
A life cycle assessment approach may be necessary to estimate the greenhouse gas emissions throughout the life cycle of a product, including production, transport of inputs (e.g. fertilizer, pesticide and feed), transport, processing, packaging and distribution of the product to retailers. The life cycle assessment is widely accepted in agriculture and other industries as a method for evaluating the environmental impacts of production and identifying the resource-intensive and emission-intensive processes within a product’s life cycle (see FAO, 2010 and 2012a).
Across climate-smart agriculture objectives of climate change adaptation and mitigation
Much of the discussion above is aimed at appraisal of climate-smart agriculture options at the local level while climate-smart agriculture potential at the policies and programmes level can also be assessed. For example, FAO developed a screening tool for use in assessing the degree to which National Agriculture Investment Plans (NAIPs) can be considered climate-smart (FAO, 2012d).
Accounting for externalities and ancillary impacts should also be considered, even if they are far more difficult to assess than improvements in adaptive capacities or the abatement of greenhouse gases. Virtually every climate-smart agriculture option will produce some positive impact (e.g. clean water or more pollinators) or negative externality and/or ancillary impact (e.g. pollution or loss of biodiversity). Whether quantifiable or not, these impacts represent real costs or benefits and should be factored into the monitoring and evaluation process.
A systematic way to analyse the synergies and trade-offs among climate-smart agriculture objectives for a large set of field level practices was developed by ICRAF in collaboration with CCAFS, FAO and the International Center for Tropical Agriculture (CIAT). The climate-smart agriculture compendium puts together all published scientific data to date on the climate-smart agriculture contributions of a large set of practices in Africa enabling meta-analyses on each objective as well as synergies and trade-offs (see Box C10.1 in module 10). Given the time and data requirements to create robust evidence on the climate-smart agriculture objectives as discussed above, climate-smart agriculture compendium can be used to provide an understanding of the synergies and trade-offs among the contributions of field level practices to climate-smart agriculture goals based on scientific evidence in similar agro-ecological and socio-economic environments.
Inadequate capacity and practicality of methods and tools
Inadequate capacities (individual, technical and institutional) and resources (human and financial) are often cited as barriers to successful assessment activities (UNFCCC, 2010b). There is a need to strengthen human and institutional capacity through training and capacity development in data collection and assessments for climate-smart agriculture (see module C1 on capacity development).
There is limited choice for appropriate analytical methods to address the specific needs and conditions of climate-smart agriculture projects for assessment. Many of the existing tools and models are intended for highly skilled technical experts in academic institutions and may not be suited for implementing climate-smart agriculture in developing countries. Further collaboration and communication between the developers of the tools and their users are necessary to ensure that simple tools that meet the needs of climate-smart agriculture practitioners are available. Some tools may be less sophisticated and produce less detailed scientific results but still meet the needs of the climate-smart agriculture community. It is necessary to find the right balance between the simplicity of the tools and the reliability of the results.